Filling in Missing Data with Low Rank Models | Madeleine Udell | WiDS 2019
About This Video
Madeleine Udell, Assistant Professor of Operations Research & Information Engineering, & Richard and Sybil Smith Sesquicentennial Fellow, Cornell University
Data scientists are often faced with the challenge of understanding a high dimensional data set organized as a table. These tables may have columns of different (sometimes, non-numeric) types, and often have many missing entries. In this talk, we discuss how to use low rank models to analyze these big messy data sets.
Low rank models perform well across a wide range of data science applications, including recommender systems, movie references, topic models, medical records, and genomics. In this talk, we introduce the mathematics of low rank models,
demonstrate a few surprising applications of low rank models in data science, and present a simple mathematical explanation for their efficacy.
In This Video
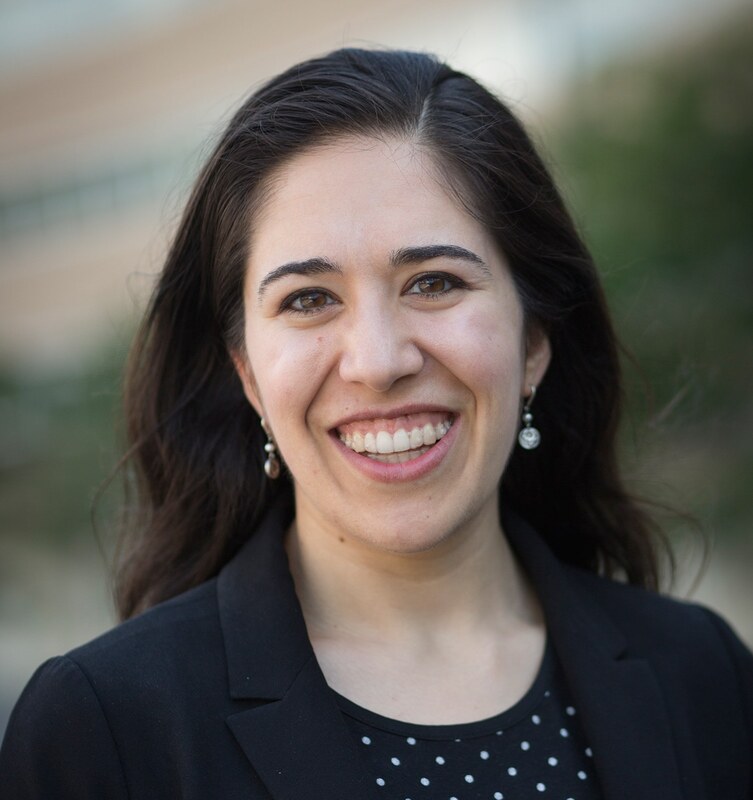
Assistant Professor, Cornell
Madeleine Udell is Assistant Professor of Operations Research and
Information Engineering and Richard and Sybil Smith Sesquicentennial
Fellow at Cornell University. She studies optimization and machine
learning for large scale data analysis and control, with applications
in marketing, demographic modeling, medical informatics, engineering
system design, and automated machine learning. She has received
several awards, including a National Science Foundation CAREER award
(2020), an Office of Naval Research (ONR) Young Investigator Award
(2020), a Cornell Engineering Research Excellence Award (2020), an
INFORMS Optimization Society Best Student Paper Award (as advisor)
(2019), and INFORMS Doing Good with Good OR (2018). Her work is
supported by grants from the NSF, ONR, DARPA, the Canadian Institutes
of Health, and Capital One.