Differential Privacy and the US Census | Cynthia Dwork | WiDS 2019
About This Video
Cynthia Dwork, Gordon McKay Professor of Computer Science at Harvard University.
Differential privacy is a mathematically rigorous definition of privacy tailored to statistical analysis of large datasets. Differentially private systems simultaneously provide useful statistics to the well-intentioned data analyst and strong protection against arbitrarily powerful adversarial system users — without needing to distinguish between the two. Differentially private systems “don’t care” what the adversary knows, now or in the future. Finally, differentially private systems can rigorously bound and control the cumulative privacy loss that accrues over many interactions with the confidential data. These unique properties, together with the abundance of auxiliary data sources and the ease with which they can be deployed by a privacy adversary, led the US Census Bureau to adopt differential privacy as the disclosure avoidance methodology of the 2020 decennial census.
This talk will motivate the definition of differential privacy, describe some of the techniques to be used in the 2020 census, and explain some of the utility gains over previous methods and the sense in which differential privacy provides a best-possible solution. Finally, the talk will highlight a few of the many remaining challenges.
In This Video
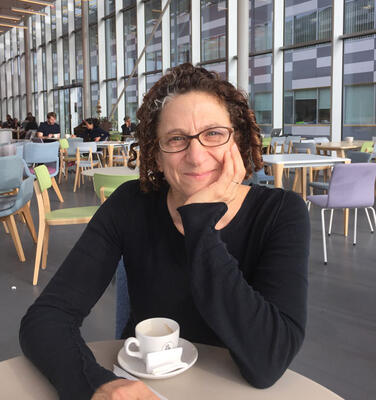
Gordon McKay Professor of Computer Science, Harvard University
Professor Cynthia Dwork is Gordon McKay Professor of Computer Science
at the Harvard University John A. Paulson School of Engineering and Applied Sciences,
Affiliated Faculty at Harvard Law School,
and Distinguished Scientist at Microsoft.
She uses theoretical computer science to place societal problems on a firm mathematical foundation.
Her recent awards and honors include the
2020 ACM SIGACT and IEEE TCMF Knuth Prize,
the 2020 IEEE Hamming Medal,
and the 2017 Gödel Prize.